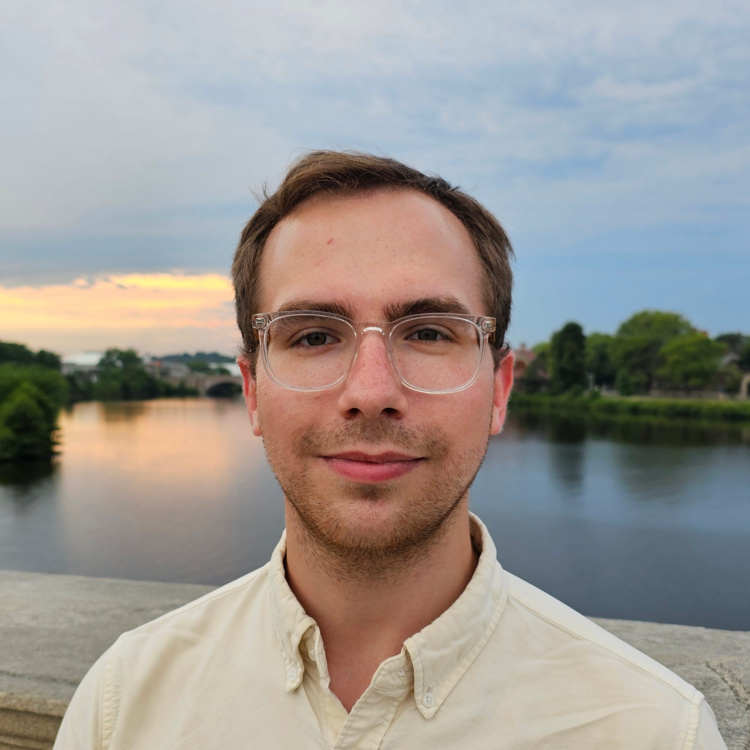
McGovern Institute Special Seminar with Jacob Zavatone-Veth
Description
Special Seminar with Jacob Zavatone-Veth
- Date: Monday, February 24, 2025
- Time: 10:00 am – 11:00 am
- Location: McGovern Seminar Room (46-3189)
Title: Mechanistic identifiability in neural circuits
Abstract: One of the central goals of neuroscience is to gain a mechanistic understanding of how the dynamics of neural circuits give rise to their observed function. A popular approach towards this end is to train recurrent neural networks (RNNs) to reproduce experimental recordings of neural activity. These trained RNNs are then treated as surrogate models of biological neural circuits, whose properties can be dissected via dynamical systems analysis. How reliable are the mechanistic insights derived from this procedure? In this talk, I will discuss some of our recent efforts to disentangle when computational mechanisms are identifiable through data-driven modeling. Focusing on the simple setting of integrator circuits, I will show how mismatches can arise both due to explicit constraints imposed by architectural choices, and due to more subtle inductive biases of learning in recurrent networks. Looking to the future, I will discuss ongoing work on model evaluation procedures that focus on mechanistic recovery.
Bio: Jacob Zavatone-Veth is a Junior Fellow of the Harvard Society of Fellows. His research is broadly focused on the theory of neural computation, with particular emphasis on how representations and dynamics are learned. He was first introduced to neuroscience during his undergraduate work in physics at Yale, where he studied visual motion detection and locomotor coordination in fruit flies with Damon Clark. He then came to Harvard for his Ph.D.; his doctoral work with Cengiz Pehlevan applied tools from statistical physics to investigate the structure of learned representations in natural and artificial neural networks. He is a recipient of a 2024 NIH Director's Early Independence Award, and of the 2024 American Physical Society Dissertation Award in Statistical and Nonlinear Physic