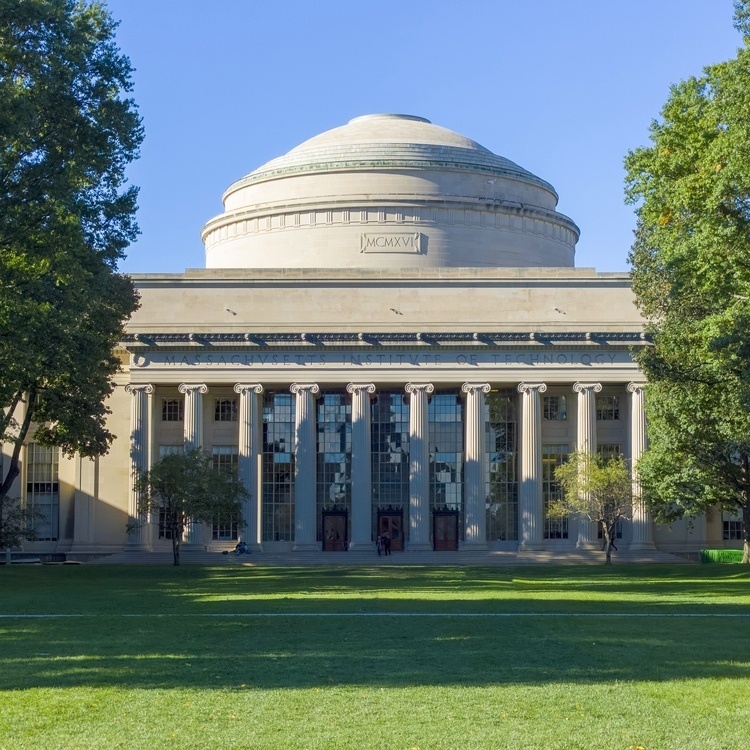
NeuroLunch: Emalie McMahon (Kanwisher Lab) & Eric Wang (Seethapathi Lab)
Description
Speaker: Emalie McMahon (Kanwisher Lab)
Title: The computational basis of human social vision
Abstract: Recent work has argued that in addition to the dorsal and ventral visual streams, there is a third visual stream projecting laterally from early visual cortex to the superior temporal sulcus that is specialized for dynamic social content. A key characteristic of the dorsal and ventral streams is hierarchical computations. I will talk about my PhD work investigating whether the lateral visual stream also has hierarchical representations of social actions using fMRI and EEG. I will also talk about early pilot data about what aspect of social interactions are represented in the STS. Together, my work provides novel insights into the neural processes that support dynamic, social vision.
Speaker: Eric Wang (Seethapathi Lab)
Title: Data-driven characterization of locomotion across rat models of ASD
Abstract: Neurodevelopmental disorders such as autism spectrum disorder (ASD) impact various aspects of cognitive and social behaviors, yet their impact on complex motor behaviors, particularly locomotion, remains poorly understood. Previous studies attempting to characterize these effects have primarily focused on isolated aspects of locomotion and have largely been limited to comparisons between a specific animal model to its neurotypical littermates. Here, we leverage a large-scale comprehensive dataset containing seven distinct ASD rat models, allowing the rats to move freely within an open arena. Utilizing 3D full-body markerless motion capture data, we aim to systematically characterize locomotion impairments across these models. In this talk, we will first review existing methods for analyzing movement patterns and then propose novel approaches that integrate domain knowledge in motor control to classify ASD subtypes. For instance, previous work has demonstrated that linear models mapping body-state estimates to foot placement exhibit variations across ASD individuals. We will compare this approach to other established methods, such as employing UMAP dimensionality reduction to visualize and benchmark movement differences. By harnessing domain-specific insights, we seek to enhance the accuracy and robustness of ASD detection and classification.