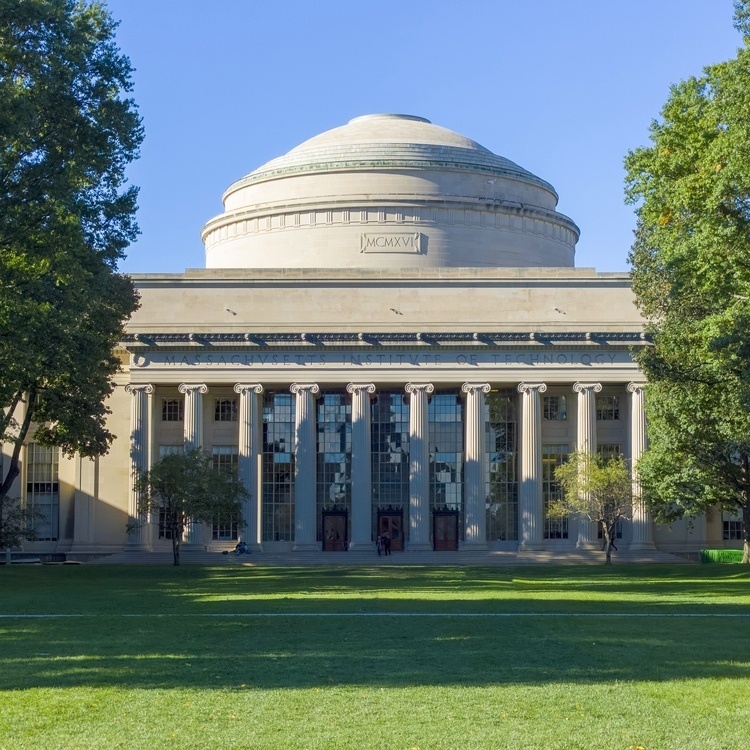
NeuroLunch: Amrita Lamba (Saxe Lab) & Laureline Logiaco (Fiete Lab)
Description
Speaker: Amrita Lamba (Saxe Lab)
Title: How credit assignment mechanisms underlie risky social decision-making in adolescents
Abstract: Adolescence is a period of rapid social development in which teens learn critical social skills by interacting with different kinds of peers. A crucial circuit known to play a role in social learning and regulation is the fronto-striatal pathway connecting the ventral striatum to prefrontal regions: ventral striatal signals communicate the value and salience of a stimulus to the prefrontal cortex which then structures this information into a usable format by binding information about value to a neural representation of the state (e.g., an individual). This coordinated fronto-striatal function thus allows the learner to differentiate between individuals and tailor one’s behavior adeptly based on social cues, a process known as credit assignment (Lamba, Nassar FeldmanHall, 2023). In this talk, I will discuss how reduced maturation of the fronto-striatal circuit alters credit assignment mechanisms in adolescents. I will first present behavioral data showing that risky social decision-making exhibited by adolescents in a trust task can be attributed, in part, to reduced credit assignment specificity in which information about the value of social partners is overgeneralized, suggesting a failure to precisely bind value-based information to the appropriate individual. I will then show neural data examining how ventral striatal functioning differs in adolescents compared to adults, in which adolescent show increased physiological responsiveness to negative social outcomes but less error-related signaling. I will then discuss interpretations of how these distinct striatal patterns may bias credit assignment towards overgeneralization, leading to risky social decision-making.
Speaker: Laureline Logiaco (Fiete Lab)
Title: Complementary goal and prediction-driven learning systems in a model of mammalian sensorimotor brain regions
Abstract: The ease with which two-legged dogs can run illustrates the versatility and agility of mammals in learning arbitrary motor skills, an ability currently unrivaled by artificial systems. How the brain supports these capacities is unclear, as control mechanisms are distributed and interdependent between many brain regions. We make progress on this question by developing a neural network model comprising three major brain systems that are known to play central roles in learned motor control: the cerebellum (CB), primary motor cortex (M1), and associative sensorimotor cortex (ASC). We leverage and modify advanced machine learning techniques to equip our model with empirically grounded computational hypotheses about how the different regions learn. The model incorporates challenging constraints that characterize real-world motor tasks: continuous space and time, no supervised motor trajectory information, and noisy, delayed, and occluded sensory input. We posit that M1 learns by exploring and reinforcing control strategies that accomplish a final goal (a policy-gradient-like algorithm), while the ASC-CB system learns through a self-supervised process relying on moment-by-moment sensory predictions. We find that the network learns realistic and robust motor strategies and that the activity in the different modeled brain regions recapitulates major features observed in a range of experiments. The three model brain systems synergize, especially in environments with very sparse observations: they take specialized roles during motor learning and execution to implement active sensing, development of a causal predictive model, and anticipatory control. The model makes varied predictions that could be tested in future experiments.