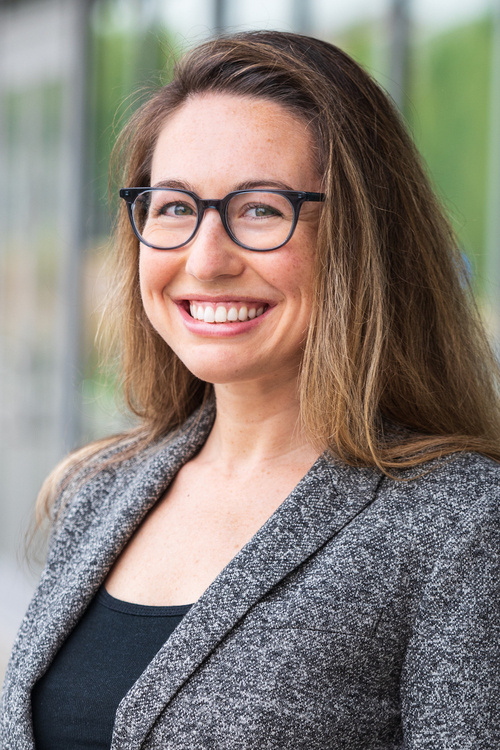
McGovern Institute Special Seminar; Rachel Ryskin
Description
Talk Title: Language comprehension adapted to the environment
Abstract: In order to understand each other across diverse contexts and situations, humans must continuously adapt their linguistic expectations. Yet, the core of their language knowledge must remain stable. Research in my lab aims to understand how humans balance flexibility and stability in language comprehension in order to efficiently exchange information in the face of variability and noise. In this talk, I will first review evidence that comprehenders learn from their environment at multiple levels including adapting to the informativity of the speaker, the probability of syntactic structures, the kinds of errors the speaker makes, and the noise in the input. I will then discuss work investigating the constraints on this continuous learning. For instance, studies with individuals across the lifespan indicate that word meanings and syntactic biases are learned on different timescales. And work with individuals with aphasia — a language disorder caused by stroke — suggests that they may not update their representations of errors in the environment as rapidly as healthy language users. I will close by discussing future directions and implications for the neural mechanisms underlying language adaptation.
Bio: Rachel Ryskin is an Assistant Professor of Cognitive & Information Sciences at the University of California, Merced, where she leads the Language, Interaction & Cognition lab. Before joining the faculty at UC Merced, she received her B.A. in Cognitive Science from Northwestern University and her PhD from the University of Illinois at Urbana-Champaign. She then completed a postdoctoral fellowship in Brain & Cognitive Sciences at the Massachusetts Institute of Technology. Work in her lab combines insights from eye-tracking, EEG, computational approaches, fieldwork, and neuropsychology to understand 1) how people use various sources of information (visuo-spatial perspective, the speaker’s knowledge state, language statistics, etc.) to infer meaning from language input that can be noisy and ambiguous, and 2) how lifelong learning allows these inferences to adapt to changes in the environment.