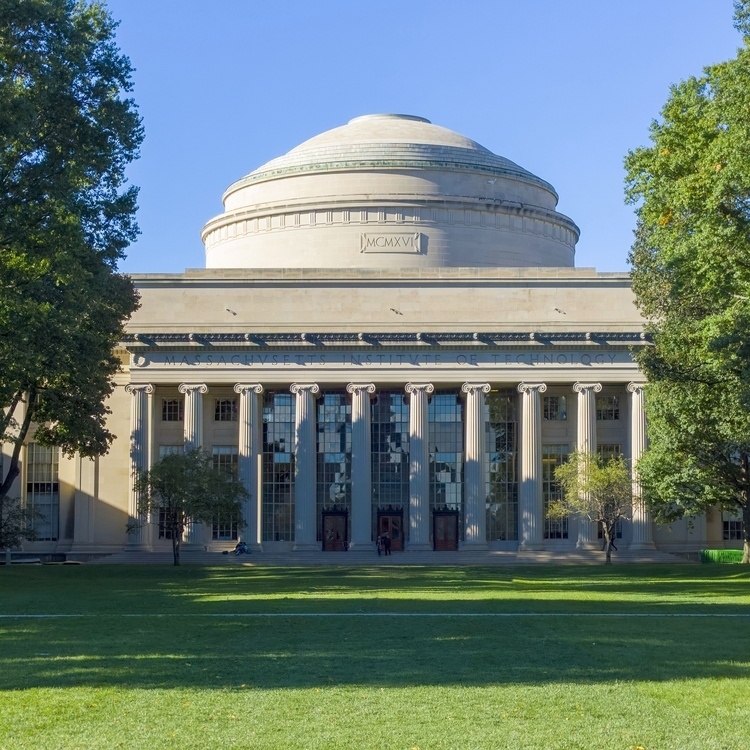
Eghbal Hosseini Thesis Defense: Towards Synergistic Understanding of Language Processing in Biological and Artificial Systems
Description
Speaker: Eghbal Hosseini
Advisor: Evelina Fedorenko
Date: Friday, April 26, 2024,
Time: 2:00pm
In-person location: Singleton Auditorium (46-3002, Third Floor of MIT Building 46)
On Zoom: https://mit.zoom.us/j/6779273385?omn=92198329747
Title: Towards Synergistic Understanding of Language Processing in Biological and Artificial Systems
Abstract: The faculty of language in the human brain relies on a network of frontal and temporal regions, predominantly in the left hemisphere, often defined as the “language network”. Despite decades of research aimed at uncovering the neural mechanisms underlying activity in this network, a computationally precise account has remained elusive. Over the past five years, artificial neural networks (ANNs) have achieved capabilities in the comprehension and production of language that are indistinguishable from those of humans, and their internal representations bear similarity to activity within the language network. In this thesis, I aim to build a synergistic understanding of language processing in both ANN models and the language network in the human brain by addressing three main questions: 1. When and how do human brains and ANN language models converge or diverge in their representations during language processing? 2. How does the amount of training data affect convergence between the human brain and ANN language models? 3. What computational mechanisms could underlie similarities in language processing between human brains and ANN language models?
To answer the first question, I demonstrate that representational spaces converge between successful ANNs and the human brain, presumably driven by the statistics of their inputs. I show that brain responses to stimuli (sentences) that are represented similarly across multiple successful ANNs are easier to predict from model representations; in contrast, brain responses to sentences that are represented differently across models are challenging to predict, despite high consistency among human participants. Extending these findings to the domain of vision, I suggest that the principle of representation universality may underlie information processing across various domains.
The second question addresses a common criticism of language ANNs: namely, that they are implausible as models of human language processing because they require vastly more training data. Using two complementary approaches, I show that ANNs can build representations similar to those in the human language network even with a “developmentally realistic” amount of training data, approximately 100 million words.
Finally, to answer the third question, I draw inspiration from computational neuroscience to reveal how ANN language models learn a predictive model of linguistic input. By focusing on representational geometry, I demonstrate that ANN models progressively “untangle” the temporal trajectory of a sentence’s representation via straightening—reduction in curvature between adjacent words as the input is passed through the model’s layers. Using this straightening mechanism, the ANN model recasts next-word prediction as a smooth linear extrapolation from the current internal state to a future state. Straightening emerges as a result of model training and scales with model size. Furthermore, the average degree of sentence straightening in the deep layers of the model correlates with corpus-based estimates of sentence surprisal, which are linked to human comprehension difficulty (e.g., as reflected in reading times).
Collectively, these lines of work provide essential ingredients for building a more computationally precise model of language processing in the human brain, leveraging synergies with artificial neural network language models.