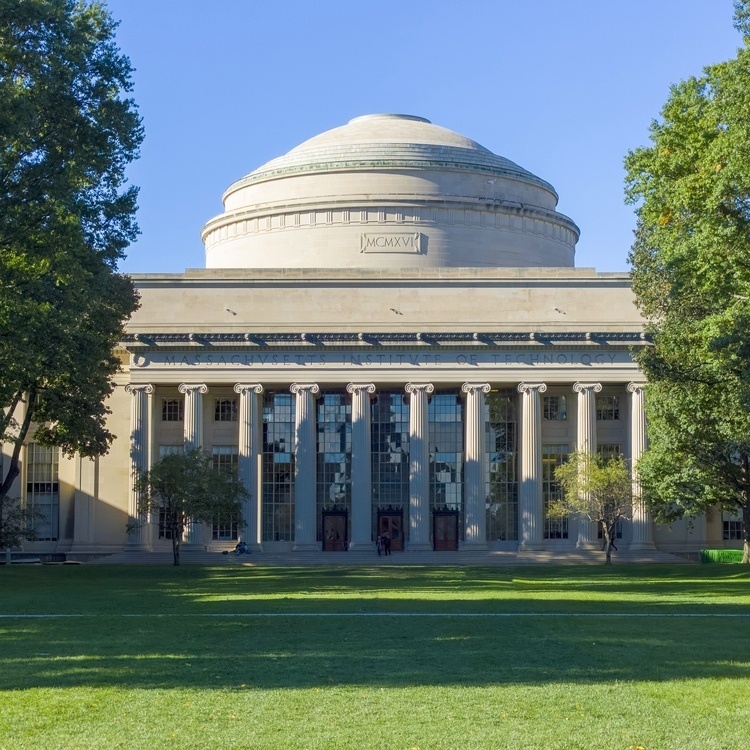
Cog Lunch: Tony Chen and Aryan Zoroufi
Description
Zoom link: https://mit.zoom.us/j/97935935903
***
Speaker: Tony Chen
Affiliation: CoCoSci (Tenenbaum Lab)
Title: Inferring the Future by Imagining the Past
Abstract: A single panel of a comic book can say a lot: it can depict not only where the characters currently are, but also their motions, their motivations, their emotions, and what they might do next. More generally, humans routinely infer complex sequences of past and future events from a static snapshot of a dynamic scene, even in situations they have never seen before. In this talk, I'll present a model of how humans make such rapid and flexible inferences, drawing on a connection between these inference problems and ray-tracing in computer graphics. Our model makes inferences that correlate well with human intuitions in a wide variety of domains, while only using a small, cognitively-plausible number of samples, outpacing prior work both in efficiency and generality.
***
Speaker: Aryan Zoroufi
Affiliation: Kanwisher Lab
Title: Cognitive, Computational, and Neural Basis of Visually-Guided Action Planning
Abstract: Human vision is a critical component of daily life, enabling us not only to recognize objects but also to infer their physical properties and plan actions accordingly. The mechanisms by which visual information guides everyday activities have long been debated, often framed within two theoretical frameworks: model-based and model-free approaches. Model-based strategies estimate the state of the world from partially-observable 2D inputs, forming beliefs about the environment and planning actions based on these beliefs. These methods typically operate by mentally simulating possible actions and selecting those with the highest expected value. While computationally expensive and relatively slow at test time, model-based approaches excel at generalizing to new scenarios without any training. In contrast, model-free approaches learn direct associations between inputs and actions —similar to end-to-end trained neural networks. Model-free strategies are often faster than model-based approaches, though at the cost of reduced adaptability to novel scenarios without extensive training. Here I propose a novel generalized approach that leverages the advantages of both methods. This hybrid strategy uses model-free approaches to suggest N possible actions, then employs model-based strategies to simulate these suggested actions and select the optimal one. I will then test which of these models (and the strategies they include) best account for human behavior during everyday actions such as finding and catching an object, by collecting behavioral measures including accuracy, reaction time, movement trajectories, and eye movements while humans perform these tasks in a virtual environment. I hypothesize that algorithms with intermediate values of N will best fit human behavior, suggesting people naturally combine both heuristic and simulation-based approaches for everyday action planning.