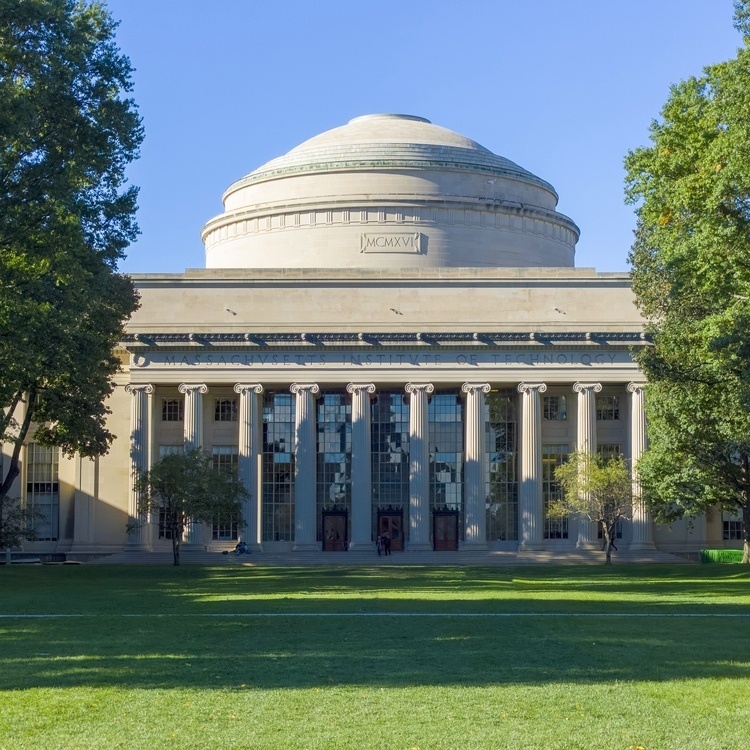
Cog Lunch: Eric Wang and Amani Maina-Kilaas
Description
***
Speaker: Eric Wang
Affiliation: Seethapathi Motor Control Group
Title: Inferring the multimodal input space of locomotor control with transformers
Abstract: Dynamic stability is a crucial component of everyday human locomotion. While we know that humans use foot placement control to walk stably, informed by errors in the body state on the previous step, we do not understand how information from multiple sensory modalities are integrated over multiple steps, and the extent to which this multimodal time-varying control is modulated across contexts. Here, we develop a machine learning framework to identify the time-varying and multimodal input space for locomotor control, and more generally, for any discrete motor output. Using our framework, we analyze how the input space of foot placement control is modulated across different environmental contexts: treadmill, overground, and uneven terrain with different terrain distributions. We find that nonlinear models outperform linear models in more complex locomotor settings by capturing nonlinear history-dependent control. Our framework enables understanding how different modalities uniquely contribute to locomotor control, thereby advancing our understanding of how complex everyday movements are controlled.
***
Speaker: Amani Maina-Kilaas
Affiliation: Computational Psycholinguistics Laboratory (Roger Levy)
Title: Large language models, human language processing, and the competence–performance distinction
Abstract: In cognitive theories there is often a distinction made between competence and performance, where competence refers to an individual’s underlying knowledge and abilities in a given domain, while performance refers to the actual execution of those abilities in real-world situations. While some challenge the need for a distinction, it can serve to help understand why something may deviate from the idealized behavior: Is the failure due to a lack of knowledge or fundamental abilities (competence), or because there are other factors preventing the demonstration of that knowledge (performance)? The distinction is broadly important in cognitive science, as many attempt to study competence, the invisible knowledge in the mind, through performance, the observables of behavior. Within linguistics, these terms refer to the idealized knowledge of language (such as grammar) and the constraints or pressures impacting real-time language use (such as working memory). The latter is the object of study in the field of psycholinguistics. As large language models (LLMs) are increasingly incorporated in psycholinguistic modeling, it becomes important to understand the exact role that they should play. LLMs are trained on vast text corpora that largely reflect human performance, yet in at least some cases their behaviors appear closer to approximating theoretically hypothesized patterns of human competence. In this early-stage work, I explore the question: How should we view LLMs with respect to this classic distinction? In what ways do they more closely resemble models of competence or of performance? Theoretically, this question is of deep importance to prediction-based accounts of human language processing, which LLMs effectively operationalize. This work aims to provide an understanding of where and why LLMs succeed and fail to model human incremental processing difficulty, shedding light on the true explanatory power of prediction-based accounts and laying the groundwork for more accurate and nuanced computational models of language.