Statistical model improves analysis of skin conductance
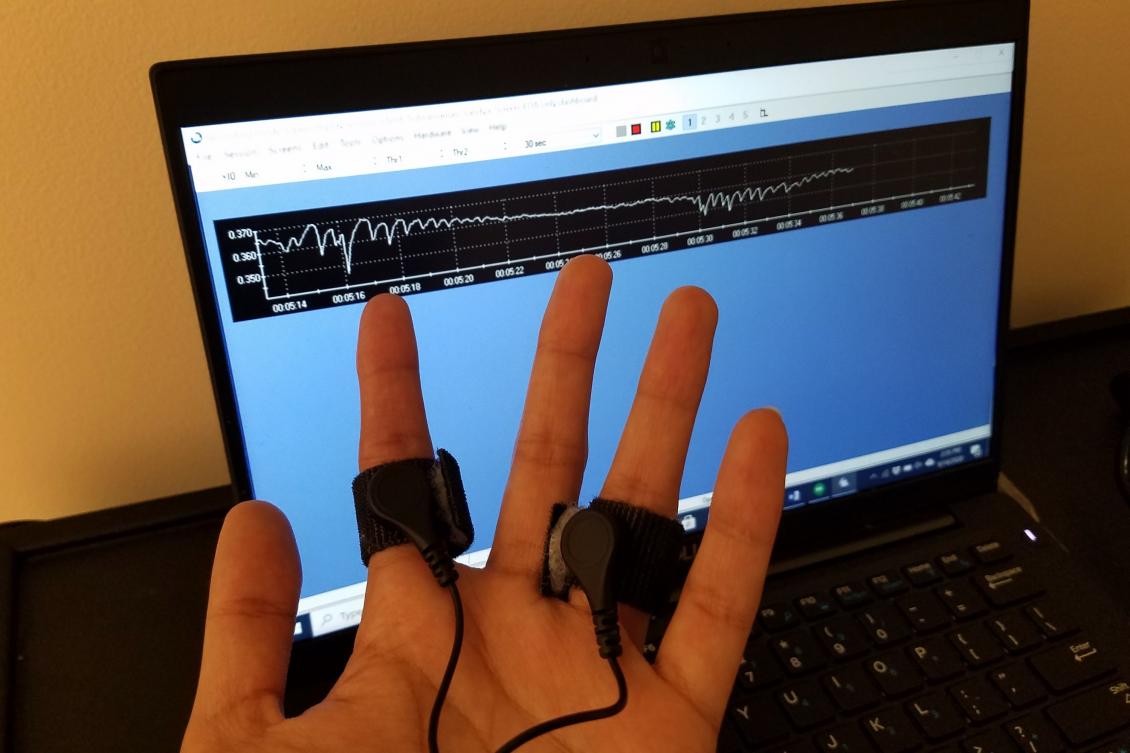
Electrodermal activity (EDA) — the sweat-induced fluctuations of skin conductance made famous in TV dramatizations of lie-detector tests — can be a truly strong indicator of subconscious, or “sympathetic,” nervous system activity for all kinds of purposes, but only if it is analyzed optimally. In a new study in the Proceedings of the National Academy of Sciences, an MIT-based team of scientists provides a new, fast, and accurate statistical model for analyzing EDA.
“Only so much of EDA is intuitive just by looking at the signal,” says Sandya Subramanian, a graduate student in the Harvard-MIT Health Sciences and Technology program and the study’s lead author. Meanwhile, existing mathematical methods of analysis either compute averages of the signal that obscure its instantaneous nature, or inefficiently force measurements into a fit with signal processing models that have nothing to do with what’s going on in the body.
To make EDA analysis faster and more accurate for interpreting internal cognitive states (like anxiety) or physiological states (like sleep), the team instead sought a statistical model that matches with the actual physiology of sweat. When stimulated by the sympathetic nervous system, glands under the skin build up a reservoir of sweat and then release it when they are full. This kind of process, called “integrate-and-fire,” is also characteristic of diverse natural phenomena like the electrical spiking of nerve cells and geyser eruptions, says senior author Emery N. Brown, the Edward Hood Taplin Professor at The Picower Institute for Learning and Memory and the Institute for Medical Engineering and Science at MIT.
A key insight of the study was the recognition that there is a well-established statistical formula for describing integrate-and-fire systems called an “inverse Gaussian” that could provide a principled way to model EDA signals.
“There is a push away from modeling actual physiology to just using off-the-shelf machine learning,” says Brown, who is also an anesthesiologist at Massachusetts General Hospital and a professor at Harvard University. “But we would have missed a very simple, straightforward, and even elegant description that is a readout of the body’s autonomic state.”
Led by Subramanian, the study team, which also included MGH researcher Riccardo Barbieri, formulated an inverse Gaussian model of EDA, and then put it to the test with 11 volunteers who wore skin conductance monitors for an hour as they sat quietly, read, or watched videos. Even while “at rest” people’s thoughts and feelings wander, creating ample variation in the EDA signal. Nevertheless, after analysis of all 11, the inverse Gaussian produced a tight fit with their actual readings.
The modeling was able to account for smaller peaks in EDA activity than other methods typically exclude and also the degree of “bumpiness” of the signal, as indicated by the length of the intervals between the pulses, Subramanian said.
In nine of the 11 cases, adding one of a few related statistical models tightened the inverse Gaussian’s fit a little further.
Subramanian said that in practical use, an EDA monitoring system based on an inverse Gaussian model alone could immediately be useful, but it could also be quickly fine-tuned by initial readings from a subject to apply the best combination of models to fit the raw data.
Even with a bit of blending of models, the new approach will be quicker, more computationally efficient, and readily interpretable than less-principled analysis methods, the authors said, because the tight coupling to physiology requires varying only a few parameters to maintain a good fit with the readings. That’s important because if the job of an EDA monitoring system is to detect significant deviations in the signal from normal levels, such as when someone feels acute discomfort, that comparison can only be made based on an accurate, real-time model of what a subject’s normal and significantly abnormal levels are.
Indeed, among the next steps in the work are tests of the model in subjects under a wider range of conditions ranging from sleep to emotional or physical stimulation and even disease states such as depression.
“Our findings provide a principled, physiologically based approach for extending EDA analyses to these more complex and important applications,” the authors conclude.
The JPB Foundation, the National Science Foundation, and the National Institutes of Health provided funding for the research.