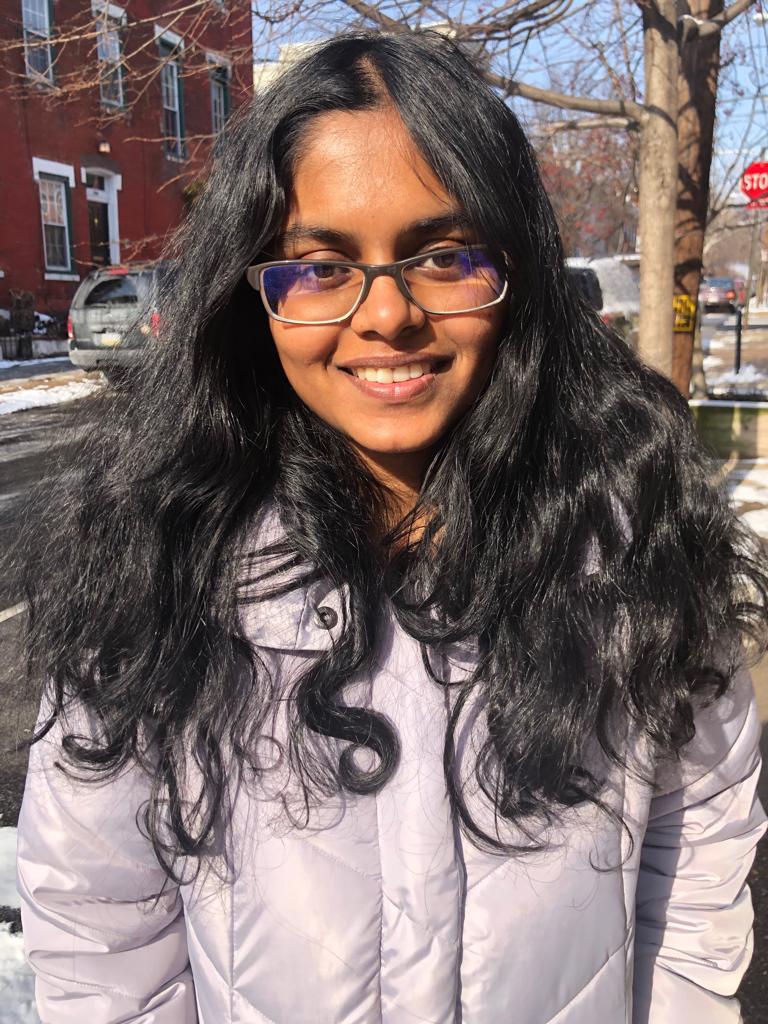
Predictive models of human motor coordination in the real world
Description
The best current robots still fall short of the versatility, efficiency, stability, and robustness to uncertainty achieved by the human sensorimotor control system. One way to understand how such remarkable movement control performance is achieved by humans is to build computational models that predict how humans perform movement tasks and learn new tasks. Existing models are either limited to detailed predictions of very simple and stereotyped movements, or produce biologically unrealistic predictions in rich environments. My research seeks to predict real-world motor coordination with parsimonious biological realism, using classical optimal control-based modeling of movement dynamics, as well as recent advances in machine learning and computer vision. In addition to being an important scientific goal, developing such predictive models will help design autonomous and robot-aided neuromotor rehabilitation.
In the first part of this talk, I will show how different optimality principles predict transient and steady-state locomotion behavior, how we used nonlinear system identification to obtain data-derived locomotor controllers, and how local reinforcement learning with stability-energy interactions explain locomotor adaptation to novel environments. In the second part of the talk, I will discuss my work on video data-driven autonomous neuromotor disorder diagnosis and artificial intelligence-based automated action feedback systems using computer vision and machine learning, leveraging data from hundreds of subjects. Finally, I will outline ongoing and future research directions on (1) building broadly predictive models of complex real-world movement and (2) making neuromotor rehabilitation autonomous and personalized. I will pursue these scientific and biomedical goals through a tight coupling between theory, computation, careful experiments, and real-world datasets.
Please use the link below to join this seminar:
Speaker Bio
Dr. Nidhi Seethapathi is a postdoctoral researcher in Bioengineering, Neuroscience, and GRASP (Robotics) Lab at University of Pennsylvania, where she works with Prof. Konrad Kording and collaborates with Prof. Michelle Johnson. Her postdoctoral work involves using data-driven techniques for modeling human movement and for autonomous neuromotor rehabilitation. She got her PhD in Mechanical Engineering from the Ohio State University in 2018. During her PhD, she worked with Manoj Srinivasan building ab initio predictive models of the human locomotion, focusing on energy optimality, stability, control, and learning. She received the selective Schlumberger Foundation Faculty for the Future Award during her doctoral studies. Nidhi received a Bachelor's degree in Mechanical Engineering from Veermata Jijabai Technological Institute in Mumbai, India.