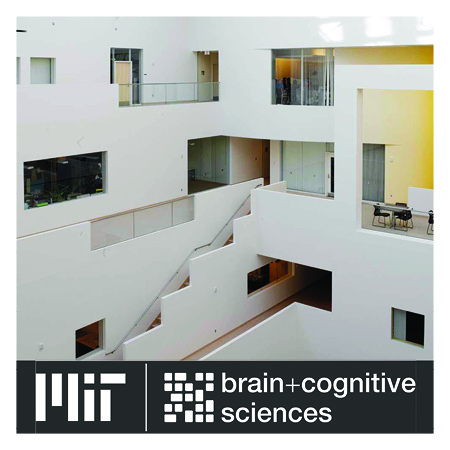
Jennifer Hu Thesis Defense: Neural language models and human linguistic knowledge
Description
Date/Time: Friday, April 14 at 2PM ET
Location: McGovern Seminar Room, 46-3189
Zoom link: https://mit.zoom.us/j/95334172487?pwd=Um9oTkpSbndUQ1ZGMCt2L0NnRzQxdz09
Title: Neural language models and human linguistic knowledge
Abstract: Language is one of the hallmarks of intelligence, demanding explanation in a theory of human cognition. However, language presents unique practical challenges for quantitative empirical research, making many linguistic theories difficult to test at naturalistic scales. Artificial neural network language models (NLMs) provide a new tool for studying language with mathematical precision and control, as they exhibit remarkably sophisticated linguistic behaviors while being fully intervenable. While NLMs differ from humans in many ways, the learning outcomes of these models can reveal the behaviors that may emerge through expressive statistical learning algorithms applied to linguistic input.
In this thesis, I demonstrate this approach through three case studies using NLMs to investigate open questions in language acquisition and comprehension. First, I use NLMs to perform controlled manipulations of language learning, and find that syntactic generalizations depend more on a learner’s inductive bias than on training data size. Second, I use NLMs to explain systematic variation in scalar inferences by approximating human listeners’ expectations over unspoken alternative sentences (e.g., “The bill was supported overwhelmingly” implies that the bill was not supported unanimously). Finally, I show that NLMs and humans exhibit similar behaviors on a set of non-literal comprehension tasks which are hypothesized to require social reasoning and world knowledge (e.g., inferring a speaker’s intended meaning from ironic statements). These findings suggest that many aspects of human linguistic knowledge may emerge through domain-general prediction mechanisms applied to linguistic forms, illustrating how an integrative approach combining theoretical, experimental, and computational methods can yield new insights into the human mind.