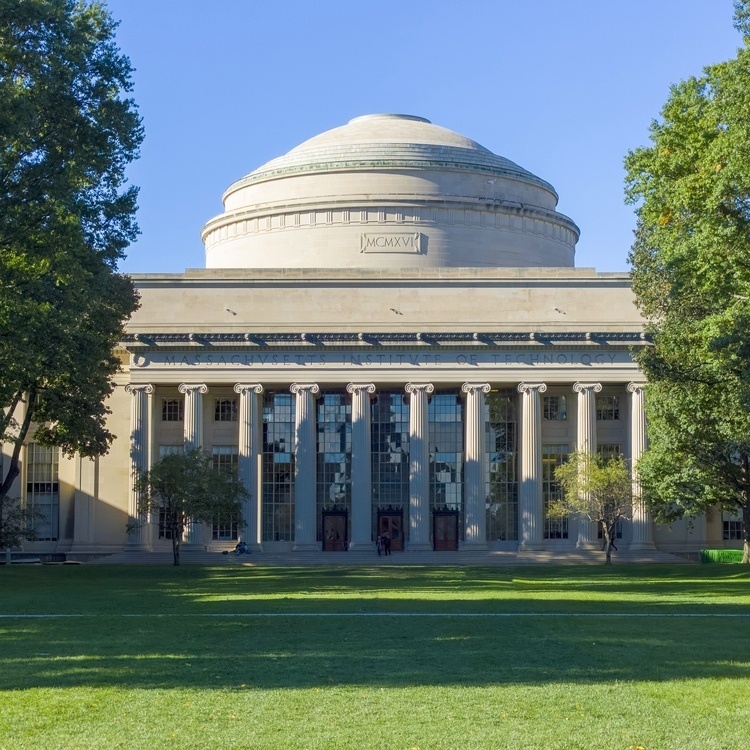
Greta Tuckute Thesis Defense: Characterizing Language Representations in the Human Mind and Brain
Description
Title: Characterizing Language Representations in the Human Mind and Brain
Advisor: Ev Fedorenko
Date and Location: Friday December 6, 11am in 46-3189 (zoom: https://mit.zoom.us/my/gretatu)
Abstract:
How do populations of biological neurons encode the meaning of a sentence?
Decades of neuroimaging research have demonstrated that regions in the left frontal and temporal parts of the brain causally and selectively support language processing (the ‘language network’). Yet, understanding the representations and computations that mediate language comprehension has proven challenging, partly due to the limited utility of probing animal models whose communication systems differ substantially from human language.
In this talk, I will first establish the use of language models as model systems for studying neural representations of language (Tuckute et al., 2024 ARN). I then investigate which aspects of a language model’s representation of the linguistic input matter most for model-to-brain similarity, showing that meanings of content words, such as nouns and verbs, matter more than syntactic structure (e.g., word order and function words) (Kauf & Tuckute et al., 2023 NOL). Second, I leverage this model-to-brain similarity to ask what kinds of linguistic input the human language regions are most responsive to. By using a language model to identify sentences that maximally drive or suppress activity in language regions, I demonstrate that these regions respond most strongly to sentences that are sufficiently linguistically well-formed but unpredictable in their structure or meaning—suggesting that this network is tuned to input predictability in the service of efficient meaning extraction (Tuckute et al., 2024 NHB). Third, I use high-field (7T) fMRI to search for the organizing dimensions of the language network. By performing a data-driven decomposition of neural responses to linguistically diverse sentences, I show that only two components—shared across individuals—emerge robustly. The first component appears to correspond to processing difficulty, in line with strong modulation of the language network by processing costs, as noted above. The second component appears to correspond to meaning abstractness. Both components are distributed across frontal and temporal brain areas but exhibit systematic topographies across participants.
Together, these projects provide a detailed characterization—across thousands of sentences and through spatially-precise neural measurements along with computational modeling—of how the frontotemporal language network supports language comprehension. I conclude by outlining potential future directions to further decipher the representations and mechanisms that underlie the astonishing human capacity to infer complex meanings through language.