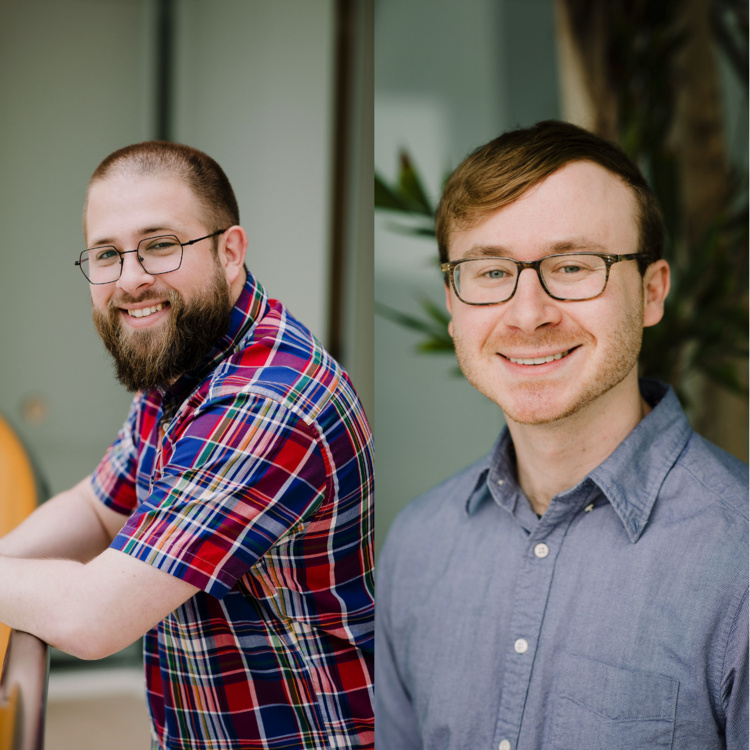
Cog Lunch: Moshe Poliak "Everyday Language Comprehension Relies on Expectations Regarding Both Structure and Meaning" & Mitchell Ostrow "Comparing the Temporal Structure of Computation in Neural Circuits with Dynamical Similarity Analysis
Description
Speaker: Moshe Poliak
Title: Everyday Language Comprehension Relies on Expectations Regarding Both Structure and Meaning: Evidence from Hindi and Russian
Abstract: Language is a tool for communication. We share the contents of our minds by putting them into code—language—and then speaking, signing, or writing it. Then, the person who hears/sees the message can decode the string of words back into meanings in their minds. However this system comes with a catch: the code may be corrupted in a host of different ways, like disfluencies in speech, background noise, or lapses of attention during perception. It has been shown that we overcome noise in language using rational comprehension: combining the perceived message with prior expectations regarding its meaning and structure. However, previous work that investigated expectations regarding sentence structure did so using rare constructions, opening up the possibility that the structural prior is only used for rarely occurring constructions, and not in everyday communication. To investigate whether the structural prior is also a part of everyday sentence processing, we use Hindi and Russian, languages that have flexible word order. That is, Hindi and Russian have several word orders that are not rare, even if one of them is more frequent than the others. In a series of studies in Hindi and Russian, we investigate how people interpret sentences that vary in plausibility and structure. We show that, in both languages participants rely both on meaning and on frequency of word order to interpret the perceived sentences, even though we did not use very rare word orders. These findings indicate that comprehenders rely on expectations regarding both structure and meaning in everyday language processing, and not only with very rare constructions.
Speaker: Mitchell Ostrow
Title: Beyond Geometry: Comparing the Temporal Structure of Computation in Neural Circuits with Dynamical Similarity Analysis
Abstract: How can we tell whether two neural networks are utilizing the same internal processes for a particular computation? This question is pertinent for multiple subfields of both neuroscience and machine learning, including neuroAI, mechanistic interpretability, and brain-machine interfaces. Standard approaches for comparing neural networks focus on the spatial geometry of latent states. Yet in recurrent networks, computations are implemented at the level of neural dynamics, which do not have a simple one-to-one mapping with geometry. To bridge this gap, we introduce a novel similarity metric that compares two systems at the level of their dynamics. Our method incorporates two components: Using recent advances in data-driven dynamical systems theory, we learn a high-dimensional linear system that accurately captures core features of the original nonlinear dynamics. Next, we compare these linear approximations via a novel extension of Procrustes Analysis that accounts for how vector fields change under orthogonal transformation. Via four case studies, we demonstrate that our method effectively identifies and distinguishes dynamic structure in recurrent neural networks (RNNs), whereas geometric methods fall short. We additionally show that our method can distinguish learning rules in an unsupervised manner. Our method therefore opens the door to novel data-driven analyses of the temporal structure of neural computation, and to more rigorous testing of RNNs as models of the brain.