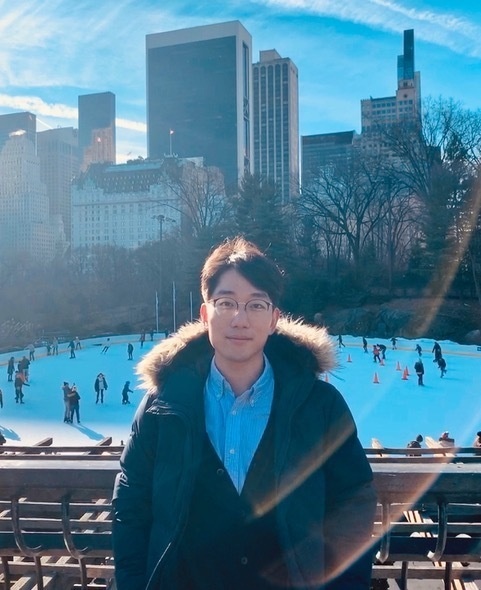
Cog Lunch: Inseung Kang "Dynamically mapping exploration and gradient-based learning during locomotor adaptation"
Description
Title: Dynamically mapping exploration and gradient-based learning during locomotor adaptation
Abstract: The ability of humans to efficiently adapt their locomotion to new environmental settings is unmatched by comparable engineered systems. Such adaptation is hypothesized to emerge as a result of two-way interactions between: 1) a low-level feedback controller that ensures stability and 2) a high-level gradient-based learner that optimizes a performance metric such as energy cost. In this talk, I will discuss our work on how the feedback controller and the learner interact to dynamically modulate movement variability in a novel environment (i.e., a split-belt treadmill). Through a model-guided analysis of the step-to-step variability, we characterize a "lumped learning parameter" that captures dynamic variations in both exploration and gradient-based learning during adaptation. We show that this parameter can distinguish learners from non-learners without directly measuring the performance metric itself -- something that conventional kinematic variability measures cannot achieve. Finally, I will discuss the implications and generalization of our findings towards developing more human-aware control of robotic exoskeletons for locomotion.
Zoom link: https://mit.zoom.us/j/8796050369