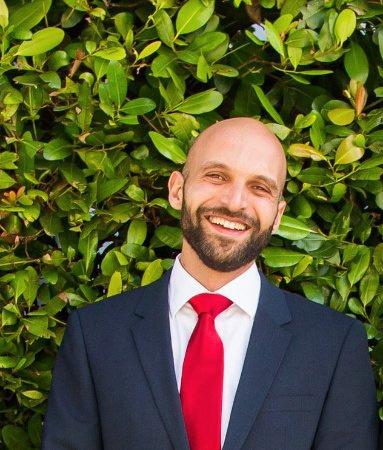
Cog Lunch: Ali Hummos "Learning Effective Cognitive Control Structures in Neural Networks through Alternating Learning and Inference"
Description
Speaker: Ali Hummos
Title: Learning Effective Cognitive Control Structures in Neural Networks through Alternating Learning and Inference
Abstract: Cognitive control is a fundamental component of human intelligence that enables individuals to adapt their beliefs and actions according to internal goals. Despite its numerous computational benefits, questions remain regarding how agents can learn cognitive control structures and when they should update these internal representations. In this work, we introduce a feedback control mechanism adapted from motor control and use it to learn effective cognitive control structures in neural networks. Our model includes a latent task embedding, and we alternate learning (updating network parameters) with inference (updating the latent embedding). We show that this algorithm can effectively learn cognitive control structures and that the resulting neural network can interact with Bayesian models. This synergistic partnership leads to feedback control signals that converge to Bayesian quantities, such as uncertainty and surprise, on previously intractable datasets. Our work represents a step forward in the development of cognitive structures in neural networks and their ability to interface with Bayesian models, increasing their relevance to human cognition.
Zoom link: https://mit.zoom.us/j/8796050369