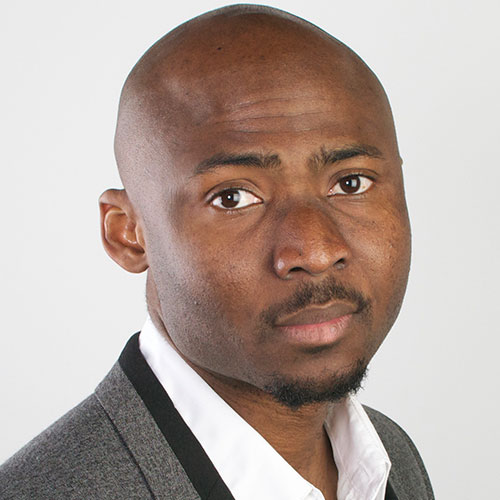
About
Lawrence Udeigwe, an Associate Professor of Mathematics at Manhattan College, joined BCS in 2021-22 as a Martin Luther King Visiting Associate Professor. Dr. Udeigwe enjoys devoting equal attention to both teaching and research, as well as being able to introduce his research material in his courses with the goal of continuously improving STEM pedagogy and, even, birthing new courses. At Manhattan College, Dr. Udeigwe introduced and created new courses in computational neuroscience and applied dynamical systems that he has taught to both graduate and undergraduate students.
In Fall 2020, Dr. Udeigwe was awarded a ~$29000 National Science Foundation research grant to serve as Senior Fellow and Visiting Scholar at the Institute for Pure and Applied Mathematics (IPAM), Los Angeles, CA, where he was one of the core participants in the long-term program Mathematical Challenges and Opportunities for Autonomous Vehicles. In 2021, he was awarded a Department of Defense research grant of $371,000 from the U.S. Army Combat Capabilities Development Command (DEVCOM) and the Army Research Office (ARO) to support his work on the homeostasis of synaptic plasticity.
Dr. Udeigwe obtained a B.S. in Mathematics and a B.A. Computer Science in 2004 from Duquesne University; an M.S. in Applied Mathematics in 2006 from the University of Delaware; and an M.A in Mathematics in 2008 and a Ph.D. in Mathematical Information Science in 2014 from the University of Pittsburgh where he wrote his dissertation under Bard Ermentrout and Paul Munro.
Outside of mathematics and science, Dr. Udeigwe is a singer-songwriter and leads the Lorens Chuno group. Among many themes, his songwriting tackles intersectionality issues faced by the contemporary African. He also explores the different ways in which mathematics and jazz can be interfaced.
Research
Synaptic Plasticity
Like many biological processes, synaptic plasticity requires a compensatory mechanism, commonly referred to as synaptic homeostasis, to help ensure that the nervous system is in a dynamic regime where it functions optimally. Synaptic homeostasis also changes and adapts to the activity of the brain. This process is called homeostatic plasticity. A review of the literature reveals a varied and somewhat paradoxical set of findings about homeostatic plasticity. While in most theoretical models, it needs to be fast -- in seconds or minutes -- and sometimes even instantaneous to achieve stability, experimentalists witness slower homeostatic plasticity in the order of hours or days. It has, in fact, been suggested that both fast and slow homeostatic mechanisms exist and that learning and memory use an interplay of both forms of homeostasis: while fast homeostatic control mechanisms (also referred to as rapid compensatory processes or RCPs) help maintain the stability of synaptic plasticity, slower ones are important for fine-tuning neural circuits.
Dr. Udeigwe’s DoD funded work uses analytical, numerical, and data-driven machine learning methods to investigate and model the dynamical interactions between synaptic plasticity and homeostatic plasticity. The overall goal is made up of three specific aims (1) to develop and analyze a multistable learning rule, with multiple firing rate setpoints, that incorporates a slow homeostasis mechanism as well two RCPs to model learning and memory formation; (2) to analyze the dynamics of -- and the possible interaction between -- synaptic plasticity and homeostatic plasticity during recurrent network activities; (3) to develop a data-driven theoretical framework in which the nonlinear evolution of synaptic activity dynamics can be approximated with a linear operator, with the secondary goal of extracting important timescale information. Investigation is done at both single-neuron and network levels and will employ a combination of theoretical tools including -- but not limited to -- stability and bifurcation analysis, perturbation analysis, data-driven spectral decomposition and deep learning of recurrent artificial recurrent neural networks.
Visual Object Recognition
By using electrophysiological recordings in animal models, it has been observed that, in all primary sensory brain regions, the firing rate of individual neurons reduces in response to a stimulus that is enlarged beyond a spatial region -- known as receptive field -- associated with these neurons, in which occupying stimuli are able to induce neuronal activity. This phenomenon is known as surround suppression, and has been studied for neurons in the primary visual cortex (V1), primary somatosensory (touch) cortex (S1), primary auditory cortex (A1), and other parts of the visual pathway . Surround suppression has been associated with a range of cognitive functions including contour integration (detecting continuity of curved and/or broken edges), motion detection, velocity detection, and texture segregation.
Dr Udeigwe’s work, in collaboration with the DiCarlo Lab at BCS, uses artificial neural networks (ANN) to investigate and further understand the mechanisms behind surround suppression and other vision normalization processes. The central idea is that, by building networks that can accomplish visual intelligence tasks, we can ask about the importance of surround suppression (a local phenomenon) in terms of overall network performance (a global phenomenon).
Publications
M.E. Bohling, L.C. Udeigwe. "The Spiking Neuron", Foundations for Undergraduate Research in Mathematics, Springer, in press
L.C. Udeigwe, P.W. Munro, G. Bard Ermentrout. "Emergent Dynamical Properties of the BCM Learning Rule." Journal of Mathematical Neuroscience. Vol 7:2, (2017), DOI: 10.1186/s13408-017-0044-6
L.C. Udeigwe and G.B. Ermentrout. "Waves and patterns on regular graphs." SIAM Journal on Applied Dynamical Systems Vol. 14(2), 1102-1129, 2015. DOI: 10.1137/140969488
D.B. Smith, L. C. Udeigwe, and J. Rubin. Physical interactions between D1 and NMDA receptors as a possible inhibitory mechanism to avoid excessive NMDA currents. BMC Neuroscience Vol. 8 (2), 2007