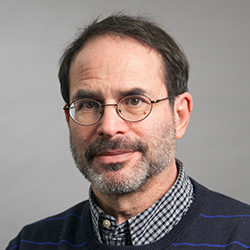
About
Edward Adelson is the John and Dorothy Wilson Professor of Vision Science at MIT, in the Department of Brain and Cognitive Sciences, and a member of the Computer Science and Artificial Intelligence Laboratory (CSAIL). He was elected to the National Academy of Sciences in 2007, and to the American Academy of Arts and Sciences in 2010. Adelson has over 100 publications on topics in human vision, machine vision, computer graphics, neuroscience, and computational photography. He is well known for contributions to multiscale image representation (such as the Laplacian pyramid) and basic concepts in early vision such as steerable filters and motion energy models. His work on layered representations for motion won the IEEE Computer Society’s Longuet-Higgins Award (2005). Adelson introduced the plenoptic function, and built the first plenoptic camera. His work on the neural mechanisms of motion perception was honored with the Rank Prize in Optoelectronics (1992). He has done pioneering work in computational photography, including the areas of image merging, denoising, and high dynamic range imaging.. He has produced some well known illusions such as the Checker-Shadow Illusion. His lab is now focused on artificial touch sensing for robotics, based on a novel elastomeric technology that allows the construction of robot fingers that are soft and sensitive like human fingers.
Research
Visual Psychophysics and Computational Vision
We study various problems in visual perception, from the standpoints of both human vision and computer vision. Current topics involve "mid-level" visual processing, including perceptual organization, as applied to motion, transparency, lightness, and texture. We are also studying the perception of materials, i.e., how it is that we can tell that something is shiny or translucent, or that it is made of plastic or metal. In much of our work, the we are interested how humans (and machines) can utilize image statistics (such as those derived from wavelet decompositions) to perform visual tasks. Some of our work has applications to image processing problems, such as image data compression, video coding, and image denoising.
Checker Shadow Illusion
The visual system needs to determine the color of objects in the world. In this case the problem is to determine the gray shade of the checks on the floor. Just measuring the light coming from a surface (the luminance) is not enough: a cast shadow will dim a surface, so that a white surface in shadow may be reflecting less light than a black surface in full light. The visual system uses several tricks to determine where the shadows are and how to compensate for them, in order to determine the shade of gray "paint" that belongs to the surface. The first trick is based on local contrast. In shadow or not, a check that is lighter than its neighboring checks is probably lighter than average, and vice versa. In the figure, the light check in shadow is surrounded by darker checks. Thus, even though the check is physically dark, it is light when compared to its neighbors. The dark checks outside the shadow, conversely, are surrounded by lighter checks, so they look dark by comparison. A second trick is based on the fact that shadows often have soft edges, while paint boundaries (like the checks) often have sharp edges. The visual system tends to ignore gradual changes in light level, so that it can determine the color of the surfaces without being misled by shadows. In this figure, the shadow looks like a shadow, both because it is fuzzy and because the shadow casting object is visible. The "paintness" of the checks is aided by the form of the "X-junctions" formed by 4 abutting checks. This type of junction is usually a signal that all the edges should be interpreted as changes in surface color rather than in terms of shadows or lighting. As with many so-called illusions, this effect really demonstrates the success rather than the failure of the visual system. The visual system is not very good at being a physical light meter, but that is not its purpose. The important task is to break the image information down into meaningful components, and thereby perceive the nature of the objects in view.
Teaching
9.357 Current Topics in Perception